
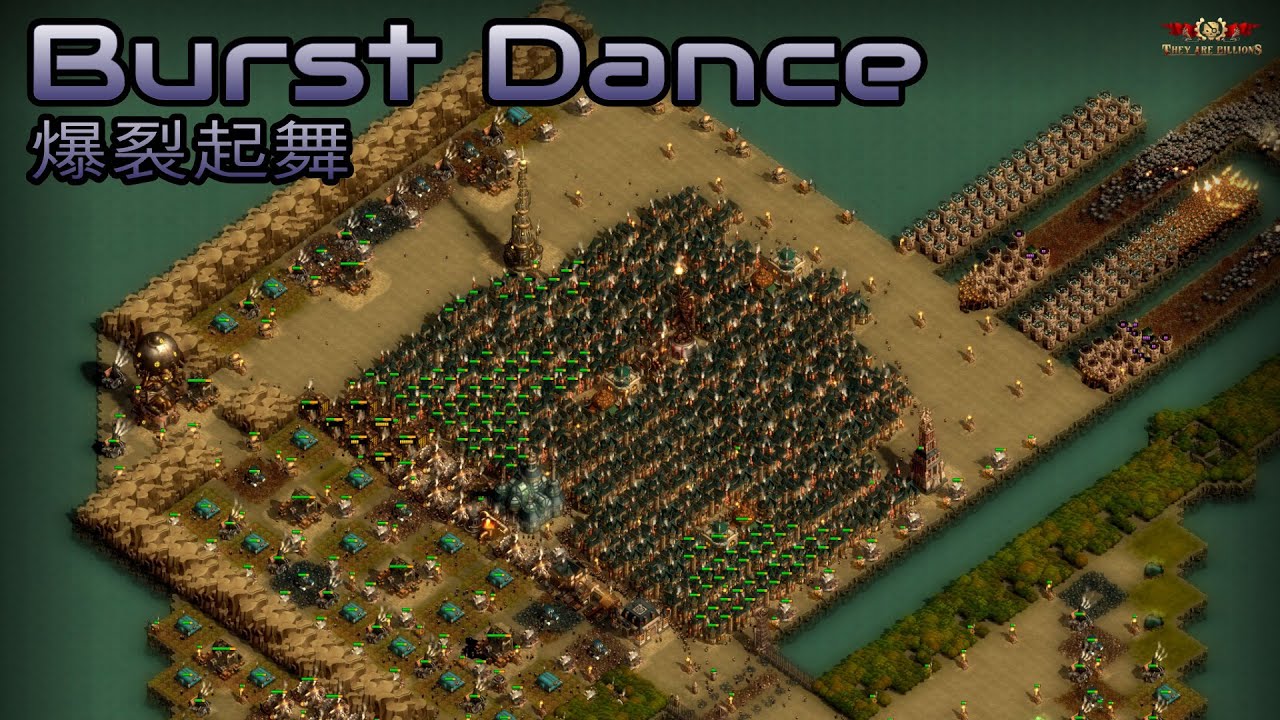
Move a few modules to the disk device if you get crashes due to a lack of RAM. Therefore, an automatically computed device map might be too intense on the CPU. While PyTorch is very good at managing GPU RAM efficiently (and giving it back when not needed), it’s not entirely true with Python and CPU RAM. infer_auto_device_map() (or device_map="auto" in load_checkpoint_and_dispatch()) tries to maximize GPU and CPU RAM it sees available when you execute it.This will be fixed in further development. While this could theoretically work on just one CPU with potential disk offload, you need at least one GPU to run this API.We are aware of the current limitations in the API: don’t put one of the first weights on GPU 0, then weights on GPU 1 and the last weight back to GPU 0) to avoid making many transfers of data between the GPUs.

To be the most efficient, make sure your device map puts the parameters on the GPUs in a sequential manner (e.g. You can also design your device_map yourself if you prefer to explicitly decide where each layer should be. Let’s download the sharded version of this model. Here is how we can use this to load the GPT2-1.5B model. If you want to use big model inference with 🤗 Transformers models, check out this documentation. It will also automatically dispatch those weights across the devices you have available (GPUs, CPU RAM), so if you are loading a sharded checkpoint, the maximum RAM usage will be the size of the biggest shard. This supports full checkpoints (a single file containing the whole state dict) as well as sharded checkpoints. The second tool 🤗 Accelerate introduces is a function load_checkpoint_and_dispatch(), that will allow you to load a checkpoint inside your empty model. Copied Īnd first_state_dict.bin containing the weights for "linear1.weight" and "linear1.bias", second_state_dict.bin the ones for "linear2.weight" and "linear2.bias" Loading weights
